Variegated bias of activity spaces
A nationwide dataset of de-identified activity spaces derived from geotagged social media data
In this article, we present a historical dataset of activity spaces, originally based on publicly posted and geotagged social media sent within the United States from 2012 to 2019. The dataset, which contains approximately 2 million users and 1.2 billion data points, is de-identified and spatially aggregated to enable ethical and broad sharing across the research community. By publishing the dataset, we hope to help researchers to quickly access and filter data to study people’s activity spaces across a range of places. In this article, we first describe the construction and characteristics of this dataset and then highlight certain limitations of the data through an illustrative analysis of potential bias—an important consideration when using data not collected through representative sampling. Our goal is to empower researchers to create novel, insightful research projects of their own design based on this dataset.
Introduction
It has been well over 15 years since location-based services (LBS) and other geo-enabled digital platforms (e.g. Facebook, Twitter) became commonplace in both daily life and research. Jumpstarting renewed interest in computational approaches in the social sciences in general (Lazer et al., 2009) and the spatial sciences in particular (Miller, 2010; Singleton & Arribas‐Bel, 2019), researchers have analyzed both the social implications of these platforms and leveraged their datasets for spatial research. This journal alone has published dozens of articles on, or using, social media data . Although this research continues, particularly during the COVID-19 pandemic (e.g. Terroso-Saenz et al., 2022), change is on the horizon. A combination of increasing privacy and ethical concerns (Zook et al., 2017), and the increasing value of data (Sadowski, 2019), means that the open platforms of the early 2000s have been largely replaced by closed APIs and walled-off data. Facebook restricts access to external researchers (Brown, 2020), and Twitter, once one of the most accessible platforms for researchers, has radically altered its API and terms and conditions after Elon Musk purchased the company in 2022.
This results in the paradoxical situation of ever-more data that at the same time is not readily accessible to the scientific community. Instead of direct API access to specific data and platforms, we increasingly encounter disembedded ‘mobile application data’ derived from people’s interactions with an opaque plethora of mobile apps. This data is generated by individual people through the digital apps they use, sold to a web of data brokers who, after aggregating and combining data from many sources, sell access to the combined data. In short, the shape of, and access to, digital data in geographic research is changing precisely as research increasingly shows the potential of this data to help understand human mobility and social processes more broadly (e.g. Ballantyne et al., 2022; Xu, 2021) . Were such data to be more widely available in open and ethical ways, more insightful research on mobility and people’s activity spaces could be conducted (Poom et al., 2020).
With this in mind, we devise a method for more widely sharing historical social media data that we have collectively created over the last decades to offer an open, standardized data source for geographic research. While a myriad of research designs might leverage social media data, we focus our effort on the concept of activity spaces specifically. Activity spaces, encompassing all the activities and locations that an individual might visit during their daily life, are a cornerstone of geographic thought tracing back to Hägerstrand’s time geography (Hägerstrand, 1970). New data sources, including the social media data described here, have enabled an increasing integration of this concept in a wide range of geographic work (Müürisepp et al., 2022). Data on activity spaces can help illuminate broader urban processes, ranging from gentrification and neighborhood change (Poorthuis et al., 2021) to segregation and access to green space (Heikinheimo et al., 2020; Väisänen et al., 2022). However, access to open data is increasingly challenging potentially leading to a fragmented landscape where studies are difficult to compare, replicate or even just start if researchers are unable to gain access to the requisite source data.
In this article, we present a historical dataset of activity spaces, originally based on publicly posted and geotagged Twitter posts across the United States from 2012 to 2019. The dataset, which contains approximately 2 million users and 1.2 billion data points, is de-identified and spatially aggregated to enable ethical and broad sharing across the research community. By publishing the dataset, we hope to help researchers to quickly access and filter data to study people’s activity spaces across a range of places, from downtown Chicago and rural Montana; or conversely to support the analysis of the origin of visitors to the nation’s national parks or one specific neighborhood in Austin, Texas. In this article, we first describe the construction and characteristics of this dataset and then highlight certain limitations of the data through an illustrative analysis of potential bias – a perennial concern when using data not collected through representative sampling (Longley et al., 2015; McNeill et al., 2017). From this basis, researchers can be empowered to create novel, insightful research projects of their own design.
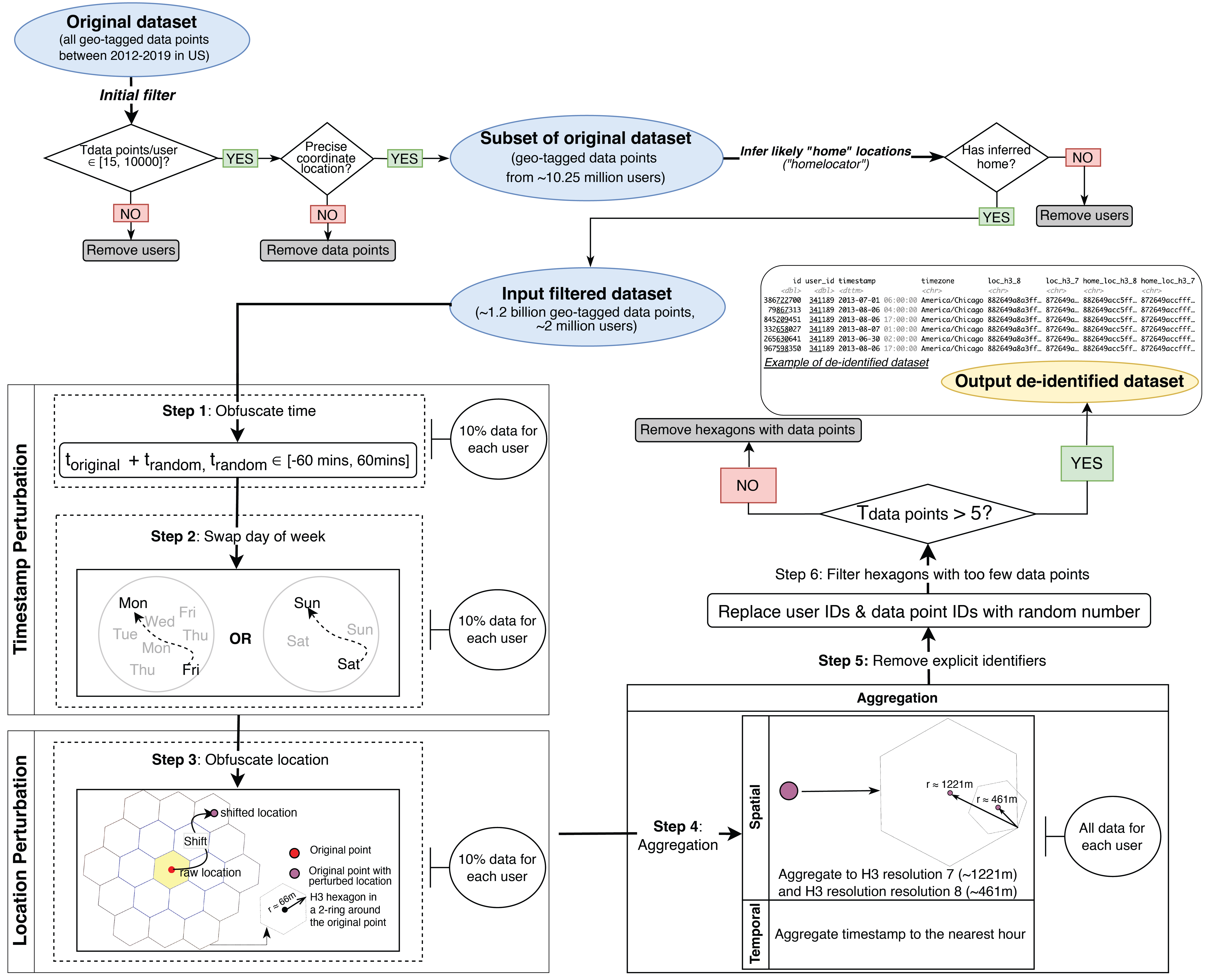
Poorthuis, A., Chen, Q. & Zook, M. (2024). A nationwide dataset of de-identified activity spaces derived from geotagged social media data Environment and Planning B: Urban Analytics and City Science, 0(0)., https://doi.org/10.1177/23998083241264051.