Decoding Smellscape
Mapping the Invisible-Decoding Perceived Urban Smells through Geosocial Media in New York City
Chen, Q., Poorthuis, A., & Crooks, A. (2025). Mapping the Invisible: Decoding Perceived Urban Smells Through Geosocial Media in New York City.Annals of the American Association of Geographers, 1-21.
Smells can shape people’s perceptions of urban spaces, influencing how individuals relate themselves to the environment both physically and emotionally. Although the urban environment has long been conceived as a multisensory experience, research has mainly focused on the visual dimension, leaving smell largely understudied. This article aims to construct a flexible and efficient bottom-up framework for capturing and classifying perceived urban smells from individuals based on geosocial media data, thus, increasing our understanding of this relatively neglected sensory dimension in urban studies. We take New York City as a case study and decode perceived smells by teasing out specific smell-related indicator words through text mining techniques from a historical set of geosocial media data (i.e., Twitter/X). The data set consists of more than 56 million data points sent by more than 3.2 million users. The results demonstrate that this approach, which combines quantitative analysis with qualitative insights, can not only reveal“hidden” places with clear spatial smell patterns, but also capture elusive smells that might otherwise be overlooked. By making perceived smells measurable and visible, we can gain a more nuanced understanding of smellscapes and people’s sensory experiences within the urban environment. Overall, we hope our study opens up new possibilities for understanding urban spaces through an olfactory lens and, more broadly, multisensory urban experience research. Key Words: geosocial media multisensory urban experiences, network analysis, New York City, smellscape, text mining, urban smells.
Introduction
Our senses–smell, vision, hearing, taste, and touch–are defined as “the faculties of perception or sensation” (OED, 2023). These multi-sensory resources on which we draw have long served as creative means to connect with each other across space and time (Finnegan, 2002). Among these, the sense of smell, which has been indicated to detect at least one trillion odors (Bushdid et al., 2014), plays a unique and potent role in providing diverse functions to humans, such as food ingestion, social communication, and the discernment of environmental odors in everyday life (Stevenson, 2010). Furthermore, the olfactory system has direct access to the amygdala and hippocampus, which are two parts of the brain responsible for emotional response and memory storage respectively. This unique neuro-anatomical structure grants the sense of smell a salient role, compared to the other senses, in the detection of emotions and retrieval of emotive memories (Herz, 2016; Igarashi et al., 2014; Prehn-Kristensen et al., 2009; Zald & Pardo, 1997). An illustrative example of this is the practical consequences of olfactory loss or dysfunction (i.e., anosmia), such as affective disturbances that includes feelings of disgust, fear, depression (Hummel & Nordin, 2005). These consequences have been shown to impact the quality of life for affected individuals, perhaps best exemplified in the recent COVID-19 pandemic, where many people have experienced the feeling of social isolation ascribed to the loss of the sense of smell following coronavirus infection (Parma et al., 2020; Pinna et al., 2020).
While smells can elicit negative emotions, they can also stimulate positive feelings that improve individuals’ health and well-being (Bentley et al., 2023). For example, the scent of blooming flowers can trigger happiness and joy, the aroma of essential oil can bring calmness and relaxation, and certain food smells can evoke nostalgic emotions that transport people back in time (i.e., the “Proust phenomenon”, Jellinek, 2004J) (e.g., Herz, 2016; Masuo et al., 2021; Porcherot et al., 2010). In urban spaces, smells have been used as important environmental cues to enrich the distinct identities of places or to bring joy and relief to people (Henshaw et al., 2016; Xiao et al., 2021). One example can be the notion of the healing garden (e.g., Silicon House–“a garden of smell”–by architects SelgasCano), which has shown its benefit for bringing restorative environmental experiences to people (Nousiainen et al., 2016).
Recently, the concept of sensorial politics (Davidson & Brash, 2021), which explores how sensory experiences are shaped by political and economic processes, has gained attention. For example, Caverly (2021) examined how unpleasant smells of abandoned buildings affected residents’ lives and how marginalized communities leveraged these experiences to influence municipal actions like demolitions. Similarly, Pennycook & Otsuji (2015) investigated the link between linguascapes and smells, highlighting that smells are crucial for understanding people’s connections to places through “spatial repertoires”—the sensory and social experiences that define the character of a place. In a sense, these sensory experiences are deeply intertwined with cultural and social values, reflecting broader societal structures and ideologies (e.g., Degen, 2008; Summers, 2021). It is this subtle and intangible intervening association among smell, emotion, and memory that impacts how we relate ourselves to the urban environment physically and emotionally. This relationship underscores the importance of analyzing and leveraging smell–an invisible (to our eyes) but rich dimension–for a better understanding and planning urban environments. Understanding how different communities perceive and experience urban smells could help planners create more inclusive and equitable urban environments, guiding interventions to improve environmental quality and residents' well-being and ensuring diverse smell experiences are considered.
However, despite its importance, smell and its complex interrelationship with humans and urban spaces has been understudied, especially compared to studies of visual senses (Henshaw, 2013; Hutmacher, 2019). One reason for this can be found in specific methodological and technological challenges. In particular, there are few off-the-shelf devices or technologies available for monitoring in-situ smells and detecting their properties, such as intensity, duration, and frequency, thus making quantitative measurement difficult (Quercia et al., 2015). Even with an actual device, it still remains difficult to collect, record and describe smells as they are generally fleeting or temporal, and can change in response to different environmental factors (e.g., climatic, temperature, wind) (Henshaw, 2013; Rodaway, 1994), resulting in an overall lack of applicable smell data (Xiao et al., 2021). Secondly, there is no consensus on how specific smells are perceived, as this can vary with culture, belief, context and personal experience (Classen et al., 1994; Majid, 2021). Together, these challenges may have led to the underestimation of smell in urban studies, particularly on how smells impact the social and emotional dimensions of cities.
So how then does one actually quantify smells in urban spaces in order to begin to understand this relatively neglected sensory dimension of urban studies? Traditionally, smells have been made tangible using methods such as smell or sensory walks (e.g., McLean, 2013, 2015, 2017; Perkins & McLean, 2020), which is generally resource-intensive and often has a limited spatio-temporal scope. As such, developing new, scalable approaches, such as those based on big data, to measure perceived urban smells holds much potential. The advent of the global position system (GPS), social media platforms like Twitter/X, and location-based services (LBS) in recent years, have opened up a host of new possibilities for social and spatial research by making it more affordable and convenient to collect spatio-temporally precise and accurate mobility data (Kwan, 2004; Tu et al., 2017). Although rare so far (Quercia et al., 2015), such data sources might help make smell collection and measurement more feasible and subsequently yield more granular insights about smellscapes (Porteous, 1985) in the context of urban studies.
This study further explores this potential by developing a methodology for the olfactory analysis of urban spaces that leverages geosocial media (i.e., Twitter) and illustrates this methodology through a case study in New York City (NYC). NYC has long been associated with unpleasant odors, especially in the 19th century (Kiechle, 2017), and due to the size and diversity of its urban fabric one would expect it to be home to a lot of different smells. Furthermore, it is one of the most prolific producers of geotagged tweets in the world (Takhteyev et al., 2012). Thus, decoding the smells of NYC provides a logical first case for an olfactory analysis with this approach.
For our analysis, we utilize a Twitter dataset that consists of around 56 million geotagged tweets sent between 2012 and 2019 in NYC. Specifically, we derive perceived urban smells and their locations from the social media posts by teasing out specific smell-related indicator words through text mining techniques. Given that smell perception is highly subjective, and individuals often experience difficulties in naming smell exactly, known as “tip of the nose problem” (Lawless & Engen, 1977). To circumvent this issue, people tend to describe smells through associations, metaphors and other indirect ways of describing smells (Rodaway, 1994). This leads to both explicit and implicit references to smells when conducting text-based analysis. For example, using Twitter, explicit conscious examples could be “stinky garbage” or “Penn Station smells like vomit”, where it is quite clear that the words are related directly to actual smell experiences. On the other hand, an implicit or unconscious example could be “On the train home, girl on other side is covered in her own vomit”. Although the term “smell” is not directly mentioned, it can be derived as the word “vomit” suggests a likely unpleasant smell experience. Figure 1 summarizes how we approach capturing both explicit and implicit smells in this research.
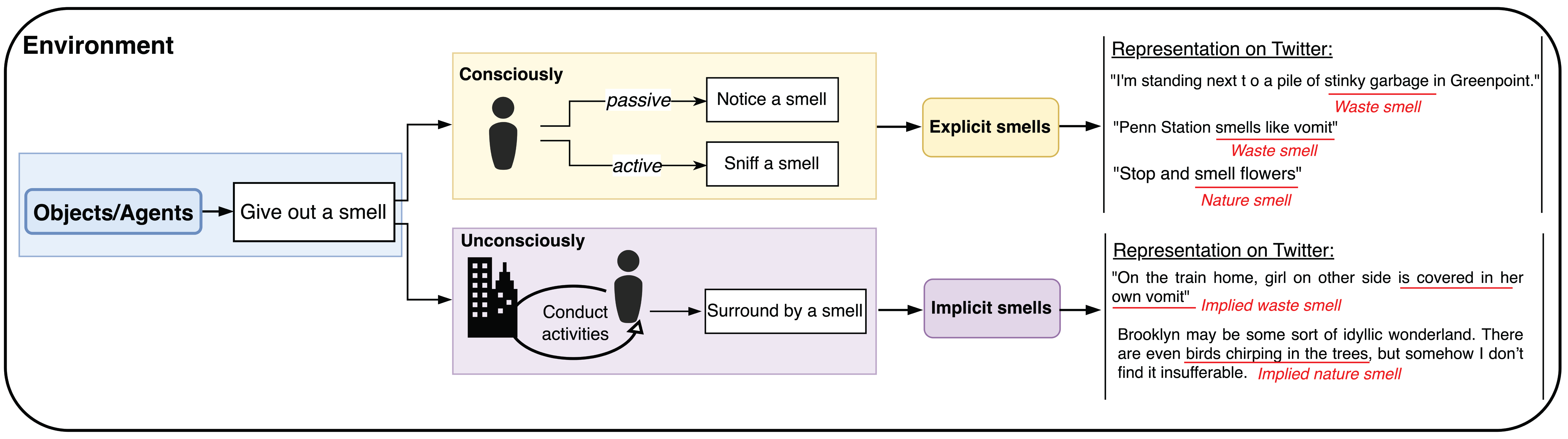
We derive both explicit and implicit smells from social media data and then use this data to analyze specific smell patterns of neighborhoods in NYC through a series of visualizations. In this way, we construct a bottom-up framework that can capture and classify perceived urban smells from individuals. The benefit of this approach is two-fold. First, it provides a flexible way to decode perceived urban smells at different scales from geosocial media data, demonstrating a proof of concept for using such data, and other platforms alike, to begin exploring the underemphasized olfactory dimension in urban studies. This can help answer questions, such as “How to actually quantify urban smells? What kinds of smells are commonly associated with different neighborhoods?” Second, our method makes the invisible smells visible and paves the way for further exploring how smells impact people’s experience of urban spaces and how they connect to urban form/functions.
To interpret and visualize the smellscape more easily, we group smells into different categories and sub-categories (see Fig.2).
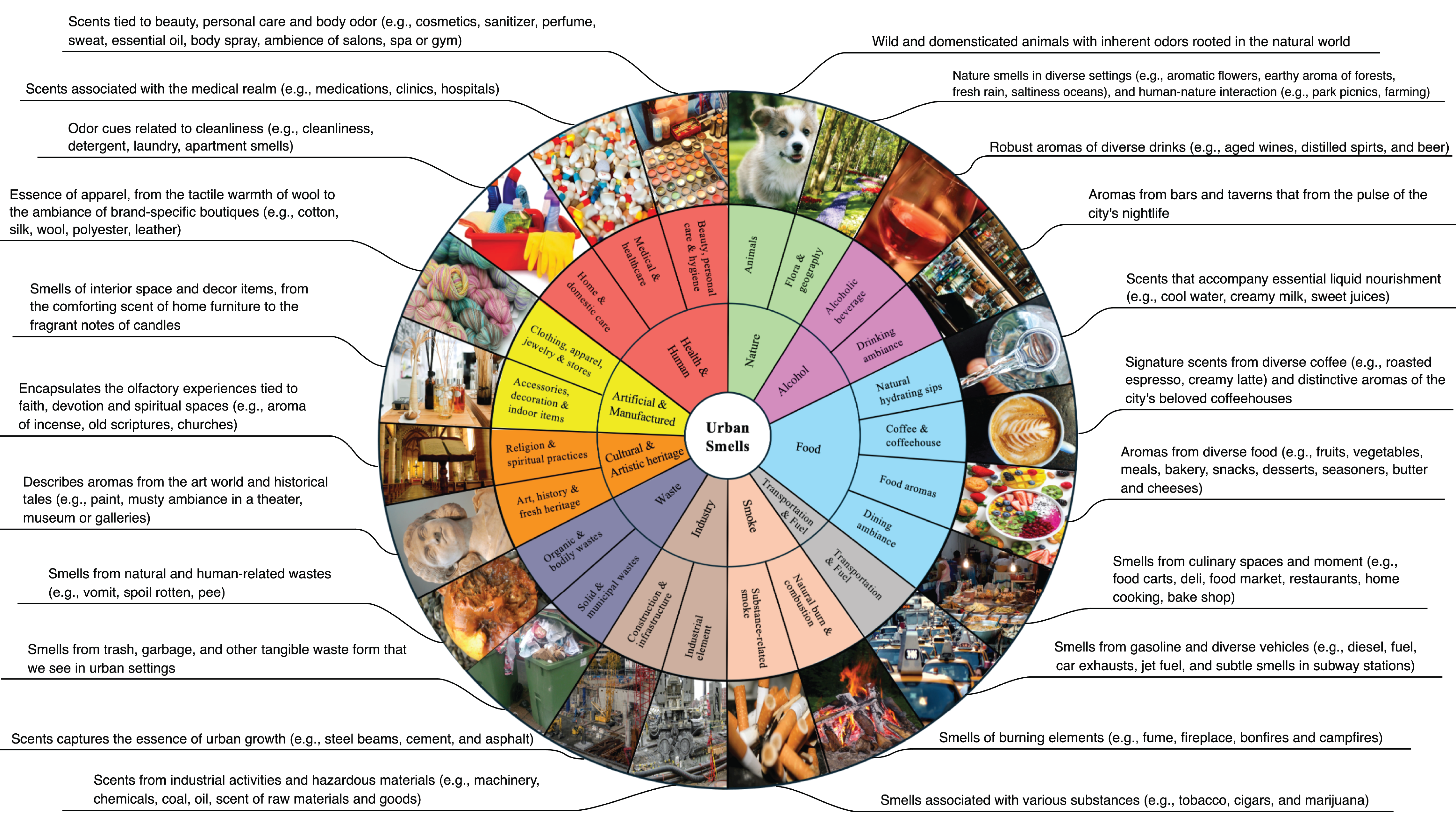
Turning to visualizing our findings, we first visualize the overall smellscape in NYC. As neighborhoods can have multiple smells associated with them, which makes visualization of the overall smellscape difficult. To address this complexity, we incorporated the weaving mapping method proposed by O’Sullivan and Bergmann (2022). More specifically, the weaving map visualizes multiple attributes simultaneously through the metaphor of woven material. It uses the concept of ‘strands’ to represent attributes. These strands interlink across boundaries, enabling multiple attributes to be reviewed simultaneously with distinct directional patterns. In Figure 4, we utilized this method to illustrate the overlaps of smell categories within each neighborhood, with each strand representing one specific smell category. Given the uneven prevalence of smell categories across the city, we have chosen to represent only the six most prevalent categories. This selective representation not only acknowledges the complex overlap of smells but also ensures clarity and effectiveness in our visual presentation.
To illustrate how the weaving map facilitates conceptual interpretation of different smells pervading the city spaces, we examine four areas (see zoom-in view in Fig.3). For instance, Area 1, near Midtown Manhattan, displays a rich “tapestry” of all six smell categories. In this area we find food (blue strands) and alcohol (purple strands) smells, likely emanating from street vendors, various restaurants and bars, reflecting a diverse and vibrant urban life. It is also characterized by major transportation hubs or intersections with heavy traffic. The proximity to the Lincoln Tunnel, which channels a constant flow of cars and buses could also contribute to the observed transportation/fuel smells (brown strands). Additionally, the present industrial (gray strands) and smoke (yellow strands) smells suggest, for example, construction activities, indicating ongoing urban development within the area. Another example–Area 3, encompasses John F. Kennedy (JFK) International Airport. Marked by significant transportation/fuel smells, it aligns with its primary urban function as a major transportation hub, yet it also intertwines with subtle hints of food and alcohol smells, indicating the airport’s eateries and bars. These examples demonstrate how different urban spaces can be distinguished by their olfactory profiles, underscoring the interaction between daily activities and urban forms and functions in shaping the city’s smellscape.
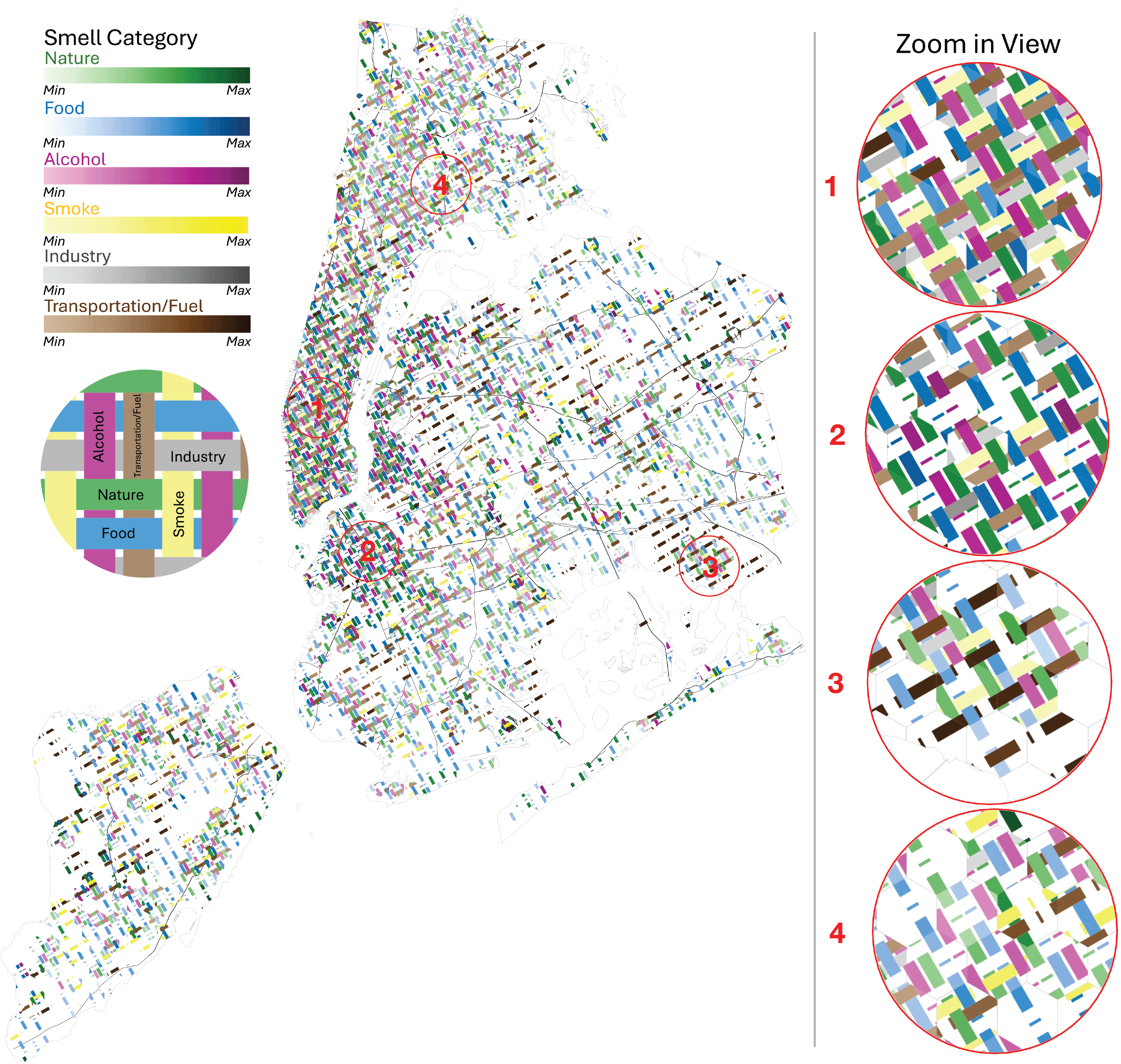
Figure 3. An overview of the six dominant overlapping smells across NYC using the weaving mapping method. The weaving map uses the concept of ‘strands’ to represent attributes. Each strand here represents one specific smell category, with the intensity of the color changing based on the density of that smell category within each neighborhood (i.e., grid cells).